- U.S.A. : 1 (470) 655-6318
- Brasil : (+55) 11-97506-0418
- Analytics
- Dic 08
Leading With Decision-Driven Data Analytics
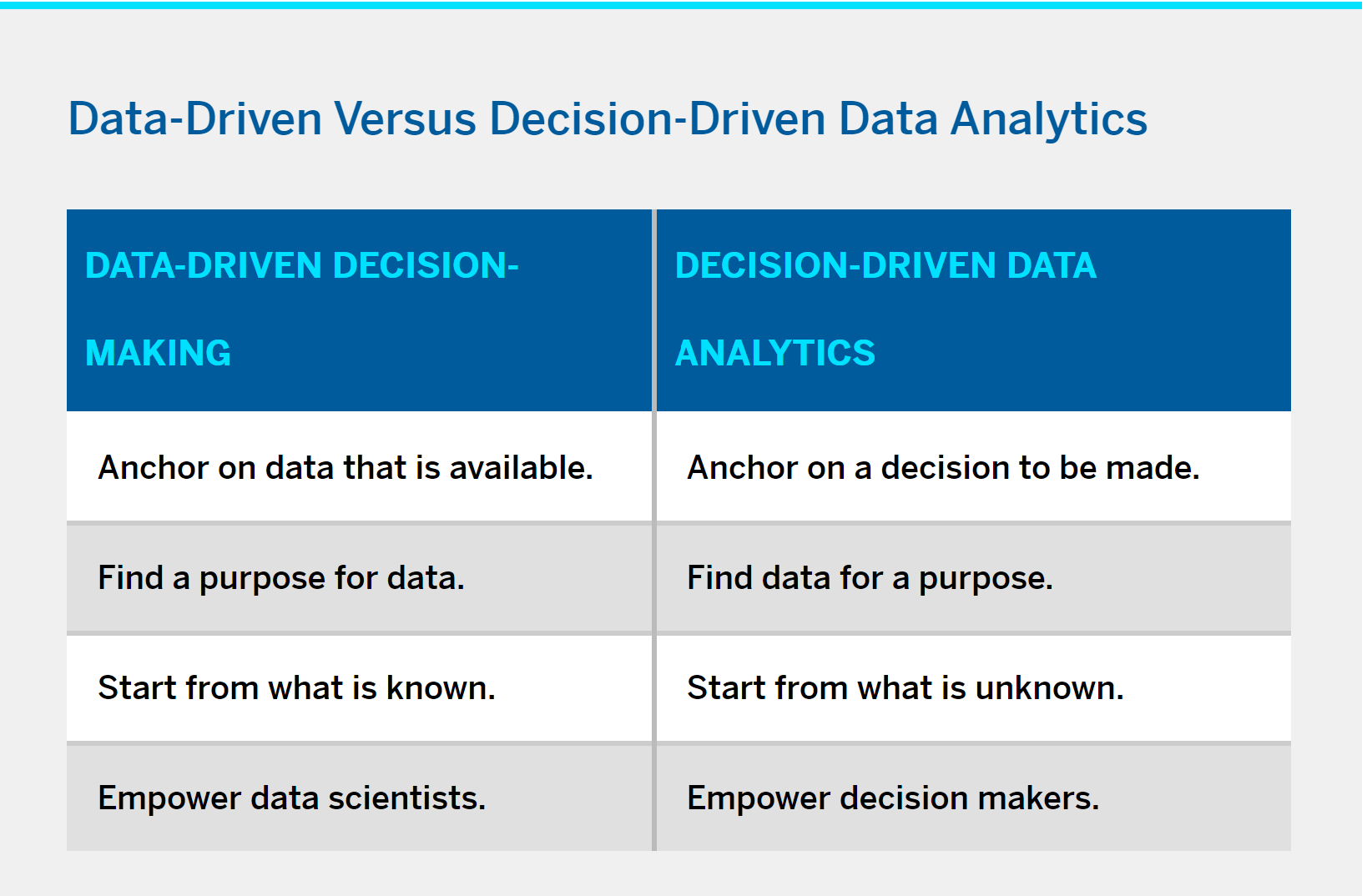
Original article available here.
Data analysts often fail to produce insights for making effective business decisions, but that’s not their fault. Leaders need to make sure that data analytics is decision-driven.
If you were to ask any major CEO about good management practices today, data-driven decision-making would invariably come up. Companies have more data than ever, but many executives say their data analytics initiatives do not provide actionable insights and produce disappointing results overall.
In practice, making decisions with data often comes down to finding a purpose for the data at hand. Companies look for ways to extract value from available data, but that doesn’t necessarily mean data analysts are answering the right questions. It’s also not a safeguard against the influence of preexisting beliefs and incentives.
The solution is simple: Instead of finding a purpose for data, find data for a purpose. We call this approach decision-driven data analytics.
‘Data-Driven’ Often Means Answering the Wrong Question
We’ll use some example companies to make our point. Let’s start with customer relationship management at RollingBoulder, a media company with a subscription-based business model. RollingBoulder’s customers can renew their annual memberships by responding to a renewal letter that they receive when their membership is about to expire. To reduce customer attrition, the organization sometimes adds a thank-you gift to these mailings.2
Over the years, RollingBoulder has developed a rich data set that describes past and current customers along various dimensions, such as location, membership duration, and website usage and behavior. The company has developed a sophisticated predictive algorithm that uses this information to quantify the likelihood that an active member will churn, and it then sends gifts to at-risk customers.
This data-driven approach to churn management is considered a best practice in the industry, but it is flawed.3 To understand why, let’s look at the central question the company is addressing with this analysis: “How likely is a customer to churn?” This is valuable information. For example, it allows the company to make projections about the value of its customer base. However, it does not address the question that is relevant here: “What is the effect of including a gift on a customer’s likelihood to churn?” This question cannot be answered based on data the company has already gathered and requires further data collection and analysis.
Data-driven decision-making anchors on available data. This often leads decision makers to focus on the wrong question. Decision-driven data analytics starts from a proper definition of the decision that needs to be made and the data that is needed to make that decision.
‘Data-Driven’ Often Means Reinforcing Preexisting Beliefs
Take the case of Gwenn & Jenny’s, an ice cream vendor that wants to know how its online advertising on Twitter impacts sales. The social media platform offers a three-step attribution process to evaluate the sales impact of advertising.4 First, a data broker, like Datalogix, shares identifying information from Gwenn & Jenny’s customers (like browser cookies, email addresses, and phone numbers) with Twitter. Next, Twitter searches for those customers in its records and, if there is a match, adds information about these customers’ activities on the platform (like whether they viewed or clicked on the brand’s tweets). Finally, analysts compare purchase decisions of customers who engaged with the Gwenn & Jenny’s brand on Twitter with purchase decisions of customers who did not.
This approach invariably reveals stark differences for a brand: Customers who saw and engaged with a brand on Twitter visit its stores more often, and they spend more on each visit. Taking the view that this data suggests social media advertising has a large impact on sales supports Twitter’s business model. It’s also consistent with clients’ beliefs that social media advertising works and that its effectiveness can easily be measured.
Twitter has sold its three-step process to many companies. However, we use it in our teaching as an example of flawed data-driven decision-making.
Comparing customers who saw a brand’s content with customers who did not see the content is like comparing apples and oranges. These customers differ in many other ways. Gwenn & Jenny’s most loyal customers are more likely to engage with the brand on Twitter, and they are also more likely to buy the brand. They don’t buy because they saw the brand appear in their Twitter feed. These loyal customers buy because they like the product, and because of this they also follow the brand on social media — not the other way around. Twitter’s approach dramatically exaggerates the impact of advertising on sales.
Data-driven decision-making empowers data providers and data scientists. The risk is that decision makers take data that is consistent with their preexisting beliefs at face value.
Moving to Decision-Driven Data Analytics
To move to a decision-driven data analytics approach, a company must start by identifying the business’s key decisions and the people who make them, and finding data for a purpose rather than finding a purpose for the data at hand. (See “Data-Driven Versus Decision-Driven Data Analytics.”)
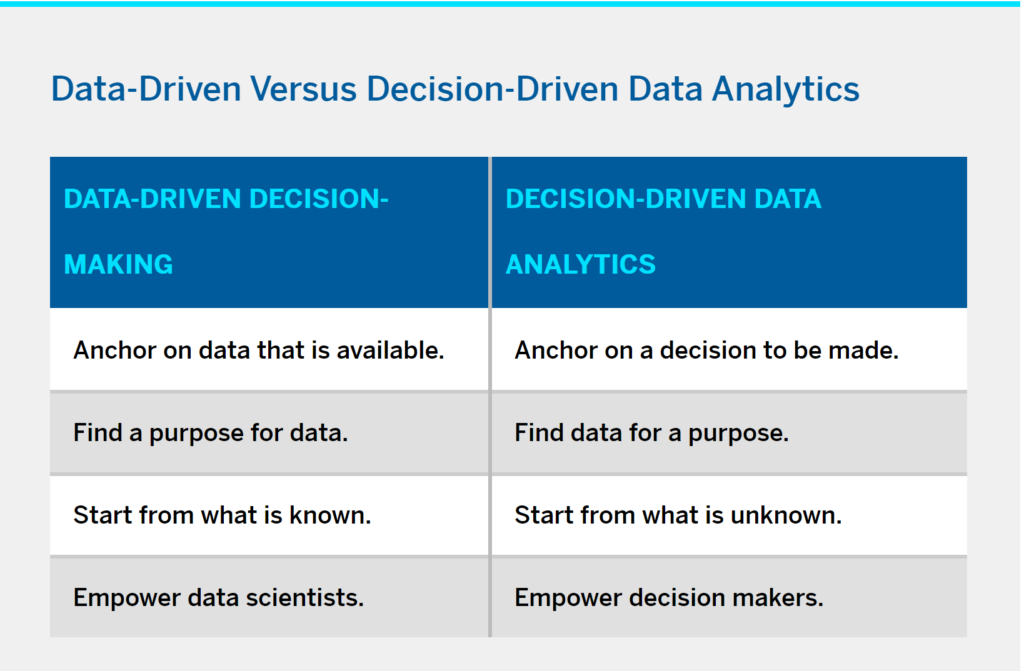
When we tell executives about the decision-driven approach, some are quick to point out a potential problem. They caution that decision makers who use data to support a decision that has already been made may be falling prey to confirmation bias. But that is not decision-driven data analytics. That is preference-driven data analytics, and it might be the worst way to make decisions — but it’s unfortunately a very common one.
To make the shift to decision-driven data analytics and avoid the pitfalls of being preference-driven, leaders can take three important steps. First, it is the responsibility of decision makers to form a narrow consideration set of alternative courses of action. Second, it is the joint responsibility of decision makers and data scientists to identify the data needed to figure out which course of action is best. The third step is to choose the best course of action.
Step 1: Identify the alternative courses of action. Decision makers should start by thinking “wide then narrow.” Many decisions are made on autopilot, after only one course of action is considered. This can harm the quality of decision-making. Thinking wide means generating many alternative courses of action. To illustrate, let’s go back to RollingBoulder. If the business objective is to increase the value of its customer base, a thank-you gift to reduce churn is just one of many possible courses of action. The company could also improve customer development (through up- or cross-selling, for example), make investments to acquire new customers (such as through sales promotions), or improve editorial content (perhaps by hiring new writers).
However, too many alternatives can make the problem intractable from a managerial and data-analytics perspective. Thinking narrow means that decision makers use their judgment to winnow courses of action. For instance, the customer relationship manager at RollingBoulder may realize that improving editorial content falls outside of her responsibilities.
By thinking “wide then narrow,” decision makers increase the likelihood that the final consideration set includes high-quality and feasible courses of action.
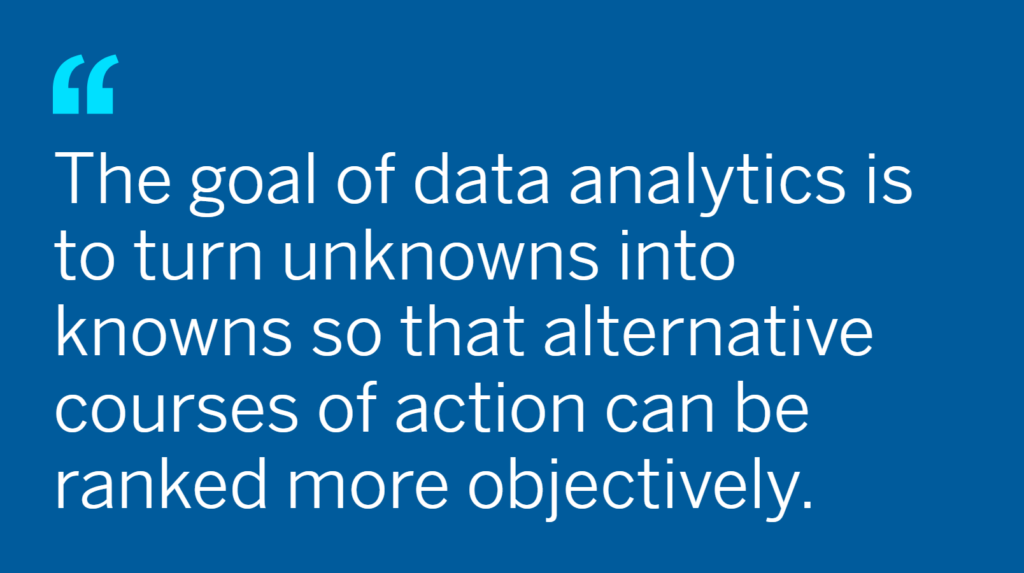
Step 2: Determine what data is needed in order to rank alternative courses of action. Decision makers and data scientists need to develop criteria to discriminate among and rank the alternative courses of action selected in step 1. The goal of data analytics is to turn unknowns into knowns so that alternative courses of action can be ranked more objectively.
Starting from the decision draws attention to unknowns, and this has a major advantage. It makes it immediately obvious that there are limits to what can be known, and that unknowns can be tackled in many different ways. For instance, if you tell people that Interbrand has determined that the Mastercard brand is worth $11 billion and ranks 57th in the world, most people will take this point estimate and rank at face value.5 If instead you ask people what they think the Mastercard brand is worth, most will realize that brand value can be quantified only imperfectly, and in a variety of ways. They are correct. Kantar Millward Brown estimates the Mastercard brand to be worth $108 billion (ranked 10th in the world), and Brand Finance estimates it at $19.8 billion (ranked 86th in the world).6 Starting from what is unknown highlights that the world is complex and uncertain.
Decision-driven data analytics is not about collecting as much data as possible. It’s crucial to consider the value of data. If you would make the same decision before turning an unknown into a known as you would after, then there is no benefit in engaging in data collection and analysis.
Oftentimes, data collected for the purpose of making a decision has more value than data that’s already available. RollingBoulder had to decide whether to add a gift to a customer’s renewal letter. To make this decision, the company needed to know how sending a gift influences a customer’s likelihood to churn. This question cannot be addressed based on the available data. It requires running a randomized controlled trial (an RCT, or A/B test): Customers are randomly selected to receive the gift or not, and the company then observes which customers churn and which customers stay.
Step 3: Select the best course of action. The final step should be easy. If the first two steps were executed well, data analytics will now reveal the best course of action.
Analyzing the data from the RCT, RollingBoulder learned an important lesson. The gift successfully reduced churn likelihood for some customers, but it backfired for others. The customers who were already least likely to churn without the intervention became even less likely to churn when targeted with the intervention. However, the customers who were most likely to churn without the intervention became even more likely to churn when targeted with the intervention. In other words, the company had always been targeting precisely the wrong customers, as do many other businesses that blindly follow the “best practice” of targeting high-risk customers.
Pablo Picasso once said: “Computers are useless. They can only give you answers.” Decision-driven data analytics emphasizes the importance of asking questions and thus the importance of managerial judgment. This approach draws attention to unknowns and the value of additional data collection and analysis. Companies and leaders who take this approach benefit by ensuring that analytics initiatives are tied to action, are focused on answering the questions that matter, and challenge rather than bolster executives’ beliefs about how the world works.
ABOUT THE AUTHORS
Bart de Langhe is a behavioral scientist and marketing professor at Esade – Ramon Llull University. Stefano Puntoni is a professor of marketing at the Rotterdam School of Management at Erasmus University and the director of the Psychology of AI lab at the Erasmus Centre for Data Analytics.
Search
Recent Posts
- How AI Can Transform Contact Centers’ Unstructured Content
- Google Brain Introduces Symbolic Programming + PyGlove Library to Reformulate AutoML
- How Call Centers Can Thrive by Successfully Managing the Unexpected with AI
- 10 Unique Use Cases for Speech Analytics
- How AWS is staking its claim on tomorrow’s contact centers
Archives
Categories
Subscribe to Our Blog
I want the latest update in...
Latest Post
How AI Can Transform Contact Centers’ Unstructured Content
- marzo 18, 2021
- [rt_reading_time postfix="mins read" postfix_singular="min read"]
Google Brain Introduces Symbolic Programming + PyGlove Library to Reformulate AutoML
- febrero 5, 2021
- [rt_reading_time postfix="mins read" postfix_singular="min read"]
How Call Centers Can Thrive by Successfully Managing the Unexpected with AI
- enero 8, 2021
- [rt_reading_time postfix="mins read" postfix_singular="min read"]
10 Unique Use Cases for Speech Analytics
- diciembre 14, 2020
- [rt_reading_time postfix="mins read" postfix_singular="min read"]
Recent Comments